Probability distributions are important in statistics because they explain the likelihood of different outcomes in a series of experiments or events. The binomial distribution is a popular probability distribution that plays an important role in statistical analysis and decision-making. Its simplicity and effectiveness make it a useful tool in a variety of fields. From quality control in manufacturing to predicting outcomes in medical testing, the binomial distribution is essential for analyzing situations with two possible outcomes: success or failure. This blog will dive deep into the characteristics of binomial distribution, formulas, and real-life applications.
What is a Binomial Distribution?
Table of Contents
One discrete probability distribution that summarizes the chances of getting a fixed number of successes in a series of independent and identical trials is the binomial distribution. It would be best if you had a constant success probability (p), a fixed number of trials (n), and two possible outcomes (success or failure). This is the binomial distribution formula:
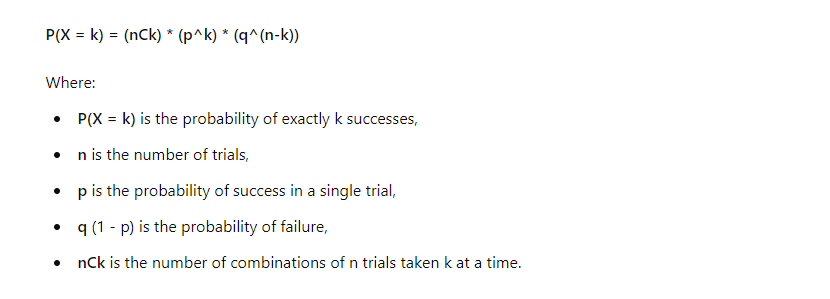
The distribution must meet certain conditions to be classified as binomial. These include a fixed number of trials, independent trials, and two possible outcomes for each trial, with the probability of success remaining constant across all trials.
Key Characteristics of Binomial Distribution
1. Fixed Number of Trials (n)
One of the unique characteristics of a binomial distribution is that the number of trials, denoted by n, is constant throughout the experiment. This means that the total number of attempts to observe a specific outcome (success or failure) is predetermined and remains constant throughout the process. For example, if an experiment requires flipping a coin ten times, the number of trials is set at ten. This consistency enables precise probability calculations because the number of opportunities for success is clearly defined.
The fixed nature of trials is essential for ensuring that the probability distribution accurately reflects the situation. Without this condition, the probabilities would become dynamic, making it impossible to calculate the outcomes with the binomial formula. Fixed trials are critical in scenarios such as quality testing, where a set number of products are inspected for flaws, and clinical trials, where a fixed number of patients are assessed for treatment efficacy. Without this fixed structure, the statistical model couldn’t make reliable predictions.
2. Two Possible Outcomes (Success or Failure)
Another key Characteristics of Binomial Distribution is that each trial has only two possible outcomes: success or failure. These outcomes are mutually exclusive and collectively exhaustive, which means that for any given trial, one of them will be successful or unsuccessful, but not both. For example, in the context of a coin toss, the two outcomes are heads (success) and tails (failure).
This binary nature is a fundamental feature of the binomial distribution, which reduces complex events to manageable success/failure conditions. In real-world applications, success does not always imply something positive; it can simply refer to the outcome of interest. In medical testing, for example, success may refer to a patient responding to a treatment, whereas failure refers to no response.
Similarly, in quality control, success could mean producing a defect-free product, whereas failure would mean discovering a defect. This clear division of outcomes allows for precise probability calculations, making the binomial distribution widely applicable in binary decision-making situations.
3. Constant Probability of Success (p)
To apply a binomial distribution, the probability of success (p) must be constant for each trial, regardless of previous successes or failures. For example, in a fair coin toss, the probability of getting heads (success) is 0.5, and this probability remains constant regardless of how many heads or tails occurred in previous tosses.
This condition of constant probability guarantees that the distribution’s outcomes are predictable and that each trial has the same chance of success. In practice, this may apply to scenarios such as a manufacturing line where the probability of producing a defective product (failure) remains constant for each item, assuming the process does not change.
The constant probability is important because it keeps the trials separate from one another, preventing previous results from influencing subsequent ones. Without this condition, the binomial formula for calculating probabilities would be invalid because the probability of each trial would vary depending on the outcomes of previous trials.
4. Independence of Trials
The independence of trials is one of the key Characteristics of Binomial Distribution, which means that the outcome of one trial does not affect the outcome of another. In other words, previous trial results have no bearing on future trials, and each trial is conducted independently. For example, in a series of ten coin tosses, the outcome of any one toss (heads or tails) has no bearing on the outcome of the next toss. Regardless of how many heads or tails have been tossed previously, the probability of getting heads remains 0.5.
Independence is an important condition because it guarantees that each trial is statistically independent, which is required when using the binomial distribution. With this independence, the results would fit the binomial model, necessitating the use of a different distribution. Independence is important in experiments where external factors do not interfere with the trials.
For example, when testing the success rate of a vaccine in different patients, each patient’s response is independent of the others, allowing the binomial distribution to predict success rates. Similarly, in product testing, each product can be evaluated independently because the quality of one item has no bearing on the next.
Mean, Variance, and Standard Deviation of Binomial Distribution
To better understand the distribution, it’s important to know its key metrics—mean, variance, and standard deviation:
- Mean (μ = np):
To get the average or predicted value, we multiply the number of trials (n) by the success probability (p). If you know the average number of trials, you may estimate the average number of successes. - Variance (σ² = npq):
With q = 1 – p representing the failure probability, the variance can be expressed as npq, which quantifies the data’s dispersion. Here, we can see how much the success rate varies from trial to trial. - Standard Deviation (σ = √npq):
A measure of the dispersion of results around the mean, the standard deviation is calculated by taking the square root of the variance. A lower standard deviation indicates that the outcomes are close to the expected value, while a higher standard deviation suggests more variability.
Real-Life Examples of Binomial Distribution
After knowing the characteristics of binomial distribution, you should also learn the real life examples of binomial distribution. Mentioned below are some Real-Life Examples of Binomial Distribution:-
Coin Toss Experiments
Repeatedly tossing a coin is one of the most basic applications of binomial distribution. For every trial, a head indicates success and a tail indicates failure. Every flip has its own unique probability and is completely separate from the others.
Quality Control in Manufacturing
The binomial distribution is a useful tool for quality control in manufacturing. If a manufacturing facility estimates a 2% defect rate for a batch of 1000 items, for instance, the binomial distribution can be used to forecast the number of defective products in that batch.
Medical Testing
The binomial distribution is often used to analyze medical test results, such as determining a treatment’s success rate. For example, if a treatment has a 70% success rate, the distribution can predict how many patients out of a given number are likely to recover.
Binomial Distribution vs Other Distributions
It is crucial to recognize when a binomial distribution is more appropriate than, say, a normal or Poisson distribution.
Binomial Distribution vs. Normal Distribution
In contrast to continuous data, which is often distributed using the normal distribution, discrete outcomes (success or failure) are best handled by the binomial distribution. In theory, the binomial distribution can be improved to a normal distribution with enough trials.
Binomial Distribution vs. Poisson Distribution
A defined number of separate trials is utilized with the binomial distribution, whereas events that happen independently across a fixed duration or region are used with the Poisson distribution. Whenever there is a steady rate of irregular events, such as calls to a customer service center, use Poisson.
Conclusion
Understanding the key characteristics of binomial distribution is essential for statistical analysis in various fields. Whether you’re managing quality control in a manufacturing process or analyzing success rates in medical trials, the binomial distribution provides a reliable way to predict outcomes based on probability.
By mastering the mean, variance, and standard deviation formulas, you can make more informed decisions and improve the accuracy of your data analysis. This distribution remains a fundamental tool for decision-making in the modern world, helping businesses and researchers optimize their operations and predictions.
FAQs
What are the main conditions required for a binomial distribution?
For a binomial distribution to be applicable, four key conditions must be met: (1) A fixed number of trials (n), (2) each trial must have only two possible outcomes (success or failure), (3) the probability of success (p) must remain constant across all trials, and (4) each trial must be independent, meaning the outcome of one trial does not influence the outcome of others.
How is the binomial distribution used in real-life applications?
The binomial distribution is commonly used in various fields, such as quality control (e.g., determining the probability of defective products in manufacturing), medical testing (e.g., evaluating the success rate of a treatment), and even simple experiments like flipping a coin. It helps analyze situations where there are repeated independent trials with two possible outcomes and a constant probability of success.