Artificial Intelligence (AI) is a hot topic these days, but did you know there are different kinds of AI? Two main categories are Generative AI and Predictive AI. These two approaches have distinct characteristics and applications, and understanding their differences (generative AI vs predictive AI) is crucial for grasping the landscape of AI technologies.
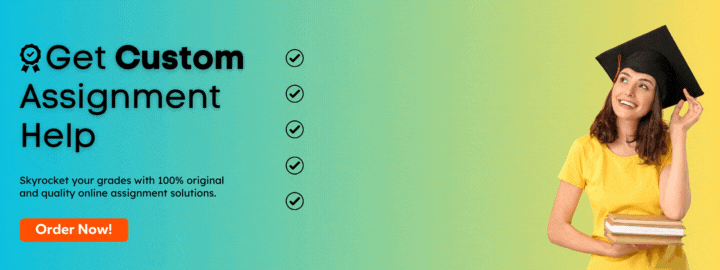
Generative AI vs Predictive AI: Detailed Differences
Table of Contents
Generative AI
Generative AI is like a creative mind in the digital realm. It has the ability to generate new content, such as images, texts, and even music, that didn’t exist before. This type of AI relies on complex algorithms and models to understand patterns in data and create something novel.
Characteristics of Generative AI
- Creativity: Generative AI makes new and unique content by learning patterns from existing data.
- Versatility: It can create different types of content, like images, videos, music, and text.
- Learning from Examples: Generative AI learns from examples and can mimic the style and characteristics of the input data.
Examples of Generative AI Applications
- Image Generation: Platforms like DeepArt and This Person Does Not Exist use generative models to create realistic images of people who don’t exist in reality.
- Text Generation: OpenAI’s GPT models can generate human-like text based on prompts provided by users.
- Music Composition: AI music composers like AIVA and Amper Music can generate original music tracks based on user preferences.
How Generative AI Works
Generative AI works by using deep learning techniques to analyze large datasets and identify patterns. It then generates new data based on these learned patterns. For example, a generative AI model trained on a dataset of human faces can generate new faces by combining features learned from the training data.
Strengths and Weaknesses of Generative AI
Strengths
- Creativity and novelty in content generation.
- Versatility in generating various types of content.
- Ability to learn from examples and mimic styles.
Weaknesses
- Difficulty in controlling the output and ensuring it meets specific criteria.
- Risk of generating biased or inappropriate content if not properly trained.
- High computational resources required for training large models.
Predictive AI
Predictive AI, on the other hand, is focused on forecasting future outcomes based on past data. It examines past data to find trends and patterns, which it then uses to predict future events or behaviors.
Characteristics of Predictive AI
- Forecasting: Predictive AI is used to forecast future outcomes, such as sales trends, customer behavior, or stock prices.
- Data Analysis: It uses statistics and machine learning to study large amounts of data and find patterns.
- Decision Support: Predictive AI provides insights and recommendations to support decision-making processes in various fields.
Examples of Predictive AI Applications
- Financial Forecasting: Banks and financial institutions use predictive AI models to forecast stock prices, detect fraud, and assess credit risk.
- Healthcare Diagnosis: Predictive AI algorithms analyze medical data to predict disease outcomes, recommend treatments, and personalize patient care.
- Retail Sales Prediction: E-commerce platforms use predictive AI to forecast customer demand, optimize pricing strategies, and manage inventory.
How Predictive AI Works
Predictive AI works by collecting and analyzing historical data to identify patterns and relationships. It then uses this information to build predictive models that can forecast future outcomes based on new data. For example, a predictive AI model trained on sales data can predict future sales trends based on factors like seasonality, promotions, and economic conditions.
Strengths and Weaknesses of Predictive AI
Strengths
- Accurate forecasting of future outcomes based on historical data.
- Data-driven insights to support decision-making processes.
- Wide range of applications across various industries.
Weaknesses
- Dependence on the quality and quantity of historical data.
- Complexity in building and maintaining predictive models.
- Potential biases in the data that can affect the accuracy of predictions.
Generative AI vs Predictive AI: Key Differences
Aspect | Generative AI | Predictive AI |
Type of Output | Generates new and original content | Predicts future outcomes based on historical data |
Focus | Creativity and novelty | Forecasting and decision support |
Examples | Image generation, text generation, music composition | Financial forecasting, healthcare diagnosis, retail sales prediction |
Strengths | Creativity, versatility, learning from examples | Accurate forecasting, data-driven insights |
Weaknesses | Difficulty in controlling output, risk of bias | Dependence on historical data, complexity |
Use Cases Of Generative AI And Predictive AI
The choice between Generative AI and Predictive AI depends on the specific task and objectives.
- Use Generative AI for tasks that require creativity and novelty, such as generating artwork, music, or text.
- Use Predictive AI for tasks that involve forecasting future outcomes or making data-driven decisions, such as financial forecasting, healthcare diagnosis, or retail sales prediction.
Challenges and Limitations
Both Generative AI and Predictive AI face challenges and limitations that need to be addressed for wider adoption and success.
Generative AI
- Control over output: Ensuring that generated content meets specific criteria and standards.
- Ethical considerations: Addressing concerns related to bias, fairness, and accountability in generated content.
- Computational resources: Managing the high computational resources required for training and running generative models.
Predictive AI
- Data quality and bias: Ensuring the quality and representativeness of historical data
to build accurate predictive models. - Interpretability: Making predictive models more interpretable and transparent to understand how predictions are made.
- Scalability: Scaling predictive models to handle large and diverse datasets and real-time applications.
Future Perspectives
The future of AI holds exciting possibilities for both Generative AI and Predictive AI.
Generative AI
- Advancements in generative models: Continued research and development in generative models to improve creativity, control, and realism in generated content.
- Use in different areas: Generative AI is being used more in entertainment, design, and education.
- Ethical rules: Creating ethical rules and regulations to make sure generative AI is used responsibly.
Predictive AI
- Advancements in predictive modeling techniques: Advancements in machine learning algorithms and techniques to build more accurate and interpretable predictive models.
- Integration with other AI technologies: Integration of predictive AI with other AI technologies such as reinforcement learning and natural language processing for more powerful and versatile applications.
- Ethical and regulatory considerations: Tackling ethical and legal issues around data privacy, bias, and fairness in predictive modeling.
Conclusion
In conclusion, Generative AI and Predictive AI are two distinct approaches in the field of AI with their own characteristics, applications, and challenges.
Understanding the differences between these two approaches (Generative AI vs Predictive AI ) is essential for choosing the right tool for specific tasks and for realizing the full potential of AI technologies in various domains.
As AI continues to evolve, it is crucial to address ethical, regulatory, and technical challenges to ensure responsible and beneficial use of AI for society.